Assessing the oceans’ resources with deep neural networks
Supervisors:
- Prof. Dr. Lars Rüpke, Helmholtz Centre for Ocean Research Kiel, Ocean Dynamics, lruepke@geomar.de
- Prof. Dr. Malte Braack, University of Kiel, Department of Applied Mathematics, braack@math.uni-kiel.de
- Prof. Dr. Mark Hannington, Helmholtz Centre for Ocean Research Kiel, Ocean Dynamics, mhannington@geomar.de
Location: Kiel
Disciplines: marine geology, applied mathematics, computer sciences
Keywords: data analysis, reduction of geological parameter uncertainties, statistical models
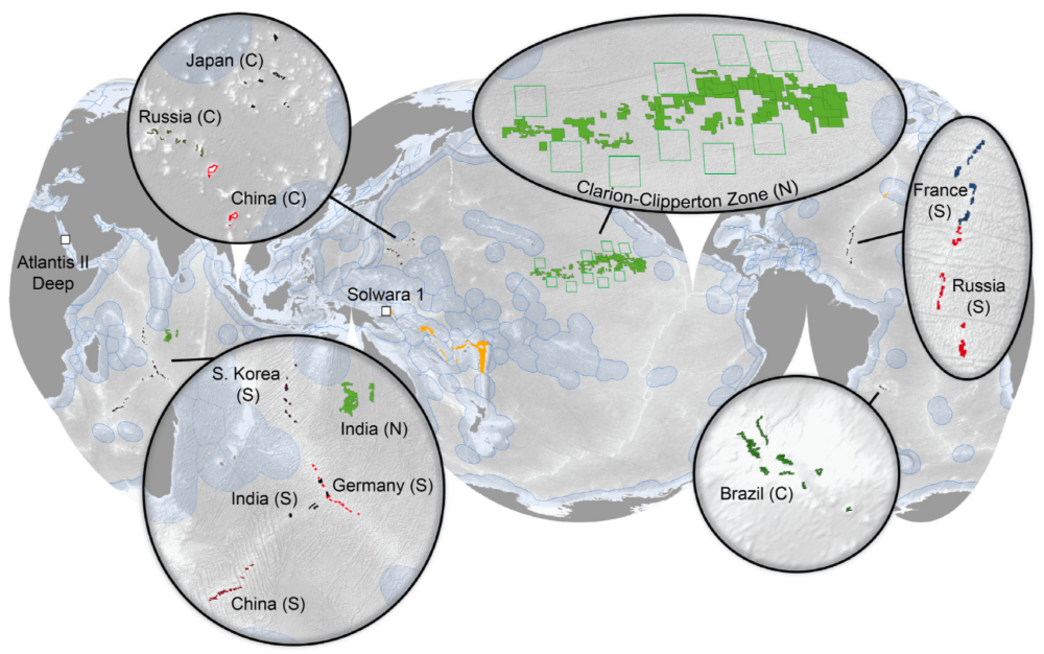
Objective: The treasure hunt for the oceans’ mineral and energy resources is on and many nations including Germany and multiple EU countries have registered claims in order to secure their national share of the presumptuously large wealth waiting to be mined from the oceans (Beaulieu et al., 2017). However, where the largest deposits are located and what the ocean’s total mineral endowment really is remains poorly known (Petersen et al., 2016). Numerical modeling has become an integral part of the exploration workflow. Petroleum and mineral system modeling involve solving partial-differential equations to simulate the key sub-seafloor processes responsible for resource formation (Hasenclever et al., 2014). Physical models are run on a so-called geomodels, which is a geometric representation of the sub-seafloor populated by properties like permeability, porosity, and rock type. Unfortunately, data is sparse in frontier areas and geomodels have large uncertainties. Modern data-science approaches try to overcome this by training statistical models in well-explored areas to make predictions in under-explored areas. While remote predictive mapping is useful to exploration companies, it remains unsatisfactory from a scientific point of view as the reason for an accumulation is not revealed, just its likeliness to exist.
Aim: We will work on a new generation of marine resource assessment models that combine data-science and physical models by adding theoretical constraints on top of the observational ones (Reichstein et al., 2019). Here deep neural networks appear to be a promising way forward as they can handle spatio-temporal dependencies, i.e. include the state of the system in addition to local environmental conditions. This is essential for predicting geological resources, which requires knowledge about Earth’s history. A particular challenge is how to include physical modeling expertise into the statistical network in order to fuse data-science with physical modeling (Chattha et. al. 2019). As a final goal we expect to gain fundamental new insights into resource formation and their global distribution. These insights are critical not only for guiding exploration but also for advising governance efforts aimed at minimizing environmental impacts of extracting mineral and energy resources.
References:
- Beaulieu, S.E., Graedel, T.E., Hannington, M.D., 2017. Should we mine the deep seafloor? Earth's Future 5, 655-658.
- Hasenclever, J., Theissen-Krah, S., Rupke, L.H., Morgan, J.P., Iyer, K., Petersen, S., Devey, C.W., 2014. Hybrid shallow on-axis and deep off-axis hydrothermal circulation at fast-spreading ridges. Nature 508, 508-512.
- Petersen, S., Krätschell, A., Augustin, N., Jamieson, J., Hein, J.R., Hannington, M.D., 2016. News from the seabed – Geological characteristics and resource potential of deep-sea mineral resources. Marine Policy 70, 175-187.
- Reichstein, M., Camps-Valls, G., Stevens, B., Jung, M., Denzler, J., Carvalhais, N., Prabhat, 2019. Deep learning and process understanding for data-driven Earth system science. Nature 566, 195-204.
- Chattha, M.A., Siddiqui. S.A., Malik, M.I., van Elst, L., Dengel, A., Ahmed, S., 2019. KINN: Incorporating Expert Knowledge in Neural Networks, arXiv:1902.05653.