Machine learning for validation and interpretation of large-scale chemical data in the ocean
Doctoral Researcher:
Martin Prinzler, University of Bremen, martin.prinzler@uni-bremen.de
Supervisors:
- Prof. Dr. Boris Koch, Alfred Wegener Institute - Helmholtz Centre for Polar and Marine Research, Bremerhaven, Boris.Koch@awi.de
- Prof. Dr. Sebastian Maneth, University of Bremen, maneth@uni-bremen.de
Additional project partner:
- Dr. Oliver Lechtenfeld, Helmholtz-Zentrum für Umweltforschung, Leipzig, oliver.lechtenfeld@ufz.de
Location: Bremen / Bremerhaven
Disciplines: statistical machine learning, marine chemistry, marine ecology
Keywords: Data fusion of chemical data, data analysis via modern machine learning methods
Motivation and Aims: Our understanding of global change and the carbon cycle is tightly linked to important chemical and biological research questions in the ocean. New chemical sensors and high-end analytical instruments in ocean research generate a plethora of novel and important data at unprecedented speed. These big datasets, which can help to answer urgent questions in marine science, create new and huge challenges with respect to data integration, processing and validation. The aim of this PhD project is to apply state-of-the-art machine learning techniques in order to automatically infer mappings between the data coming from different analytical sensors and devices. Based on existing training data, the project aims at predicting the chemical composition of test samples according to their origin. For instance, by “forensic” evaluation of existing chemical fingerprints derived from thousands of mass spectrometric analyses, the origin of organic matter in ocean water shall be predicted. To achieve this goal, we will engage with new measuring devices; for instance, a recently developed in situ fluorescence sensor. In ocean excursions, the PhD candidate will also take samples and measure in situ data, which can then be directly applied within the machine learning pipeline to make predictions. Our data will be enriched and integrated using several other low level sensors for location, depth, temperature, light, and others. The PhD project aims at providing a novel data analysis front end, which runs in any web browser and which can swiftly be applied by other researchers to tap into our existing data collections, or, to upload their own new data for analysis.
Objectives: (1) Implementation of an innovative machine learning pipeline in chemical oceanography (2) Implementation of a slick user front end for large scale analysis of marine chemical data, e.g. based on (Leefmann, Frickenhaus, Koch, 2019). (3) Experimental evaluation of the ML pipeline using state-of-the-art classification methods (such as random forests, support vector machines, neural networks, and others) over existing and new datasets acquired during the project.
References
Arroyuelo, D., Claude, F.,Maneth, S., Mäkinen, V., Navarro, G., Nguyen, K., Sirén, J., Välimäki, N. (2015). Fast in-memory XPath search using compressed indexes. Softw., Pract. Exper. 45, 399-434
Dubinenkov I., Flerus R., Schmitt-Kopplin P., Kattner G., Koch B.P. (2015). Origin-specific molecular signatures of dissolved organic matter in the Lena Delta. Biogeochemistry, 123, 1–14.
Koch, B. P., Witt, M., Kattner, G., Dittmar, T. (2017). Fundamentals of molecular formula assignment to ultrahigh resolution mass data of natural organic matter, Analytical Chemistry 79, 1758-1763.
Leefmann T., Frickenhaus S., Koch B.P. (2019). UltraMassExplorer - a browser-based application for the evaluation of high-resolution mass spectrometric data. Rapid Communications in Mass Spectrometry 33, 193-202.
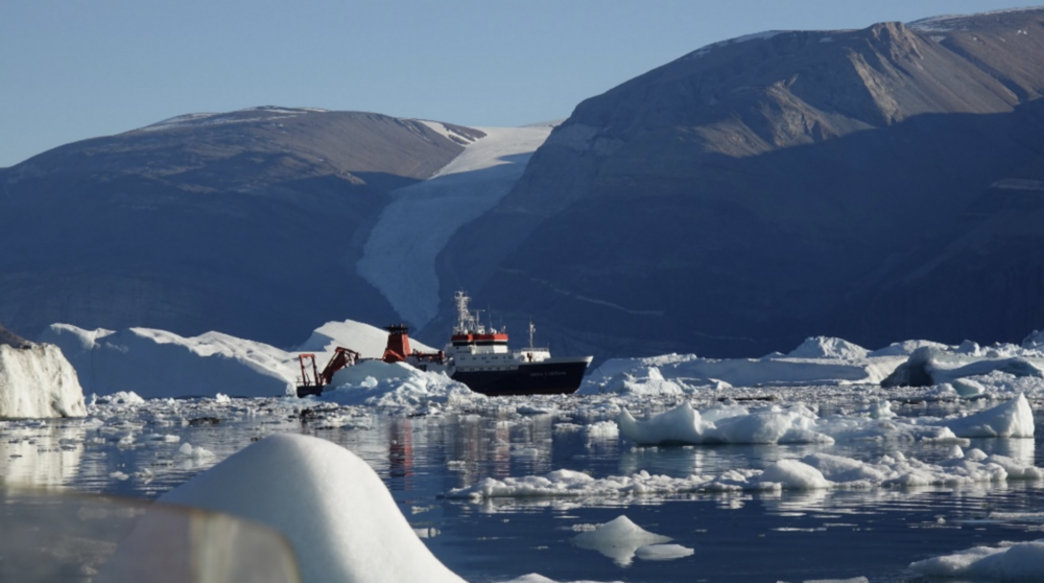